Introduction
Radars have been one of the key elements in the development of modern industrial applications. Initially used for military purposes during World War II, radars have expanded their use to numerous civilian applications, such as space exploration, weather monitoring, air traffic control systems, and various automotive applications. Currently, radar signal processing represents an ever-expanding field with multiple development opportunities, especially in the automotive industry. With the rapid advancement of autonomous driving assistance systems (ADAS) and autonomous vehicles, the accurate scanning of the environment and radar-based object detection and classification have become crucial to deploy safe L2+ AD applications.
As the primary sensor for car applications such as advanced emergency braking systems and lane-keeping, radars have become a valuable automotive component that ultimately involves the safety of human lives. However, the amount of car sensor data needed to be processed in real-time is rapidly increasing. Likewise, the precision required for reliable classifications requires more data points per frame and sub-1-degree angular resolution. Additionally, there is also the challenge not to linearly increase the computational requirements within the car when processing all this concurring data. Given this situation, the question arises: How to achieve better, trustworthy radar detections more efficiently? The answer lies in the capabilities of machine learning, which is pushing the performance of radar systems for the automotive industry to new frontiers.
Current radar signal processing
Radars can identify targets by scanning the trajectory and measuring the distance of any object, which generates a position of that object with respect to the radar. Modern radars can employ different technologies to produce signals and create classifications. On the one hand, pulse-Doppler radars are utilized in longer-range radar detections, from one to dozens of kilometers. Similarly, frequency-modulated continuous-wave (FMCW) is considered slightly more accurate than pulse and is preferred for other challenging applications. These two radars options can work on different frequency bands for automotive use, either 24/26 GHz or 77/79 GHz. Accordingly, radars can also be classified depending on their measuring range, as a Short-Range Radar (SSR, up to 50m), Mid-Range Radar (MRR, up to 100m), and Long Range Radar (LRR, up to 250m).
Distinctive detection mechanisms can perform with radar signals to enable precise detections. One of the most widely accepted automobile applications today is constant false alarm rate (CFAR) which uses a common form of adaptive algorithms to identify targets amid noisy environments, clusters, and other interference. Put simply; false alarm rates recognize erroneous radar target detections caused by interfering signals exceeding the detection threshold. Consequently, a constant false alarm rate scheme deviates the detection threshold in the function of the sensed environment. The process estimates the level of interference in the radar range and uses this measure to resolve if there is a target in the radar spectrum.
The advantages of this method are a relatively low target loss and better effectiveness of the threshold preparation. The disadvantages are that two adjacent targets can overlap, leading to limited or erroneous detections in difficult multi-target situations and the high processing power required to execute the sorting algorithm, engrossing the system's computing requirements
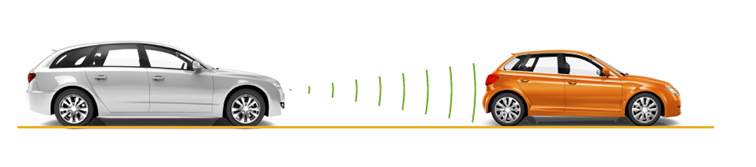
Another similar system that rely on the electromagnetic spectrum are LiDAR, using infrared laser lights instead of radio waves. LiDAR systems can detect an object within a range of 30 to 200 meters with high accuracy in terms of scope, resolution, and position. Although LiDAR’s have become more popular among OEMs, the limitations of this system become visible when signals are attenuated at long distances or when trying to recognize objects in its vicinity with limited weather conditions. Moreover, the very high prices for these sensors do not make them cost-effective for mass production.
Hence, radar technology has proven to be one of the most reliable, non-contacting forms of sensing for the automotive industry as they provide accurate, reliable measures. Radars are less sensitive to weather phenomena such as fog, rain, or snow that could hinder the visibility of systems like LiDAR or cameras. Radio waves can perform under these weather conditions without losing accuracy. Moreover, automotive radars' detection range can be as short as a few up to two hundred meters, with good resolution and position estimations. Ideally, the combination radar-camera is more desirable as the camera's weaknesses are offset by the radar's strengths and vice versa.
However, despite all the benefits of radar automobile systems, it is essential to consider that to obtain the previously mentioned results, signal systems require the transmitter and receiver of the radar to work simultaneously on separate antennas. The short duty cycles entail high digital sample rates, elevated digital signal processing capabilities, and very high-speed analog pulsing circuitry, which is not always feasible in low-cost automotive systems. Also, automotive radar systems require low latency processing, which adds up processing needs to be performed in parallel. Even if the computational capacity is resolved, complex angle measurement and target classifications hinder the potential of radar for fully AD applications.
Unlocking better detections with artificial intelligence
So, given the limitations of current radar systems in terms of computational capacity and target classifications, what are the alternatives to propel even safer driving applications? The answer lies in artificial intelligence. Modern radar applications can utilize machine learning algorithms to work on raw data. Edge-AI can play both a denoising and a cognitive role in dissecting information from the radar. This approach has the potential to achieve better detections and enhance the safety of automotive applications at lower costs.
The value for autonomous and ADAS applications lies in the quality and density of data points of individual detections. Put differently, if one does classification on objects with more data points it will simply lead to better detection rates and more accurate classification.
At Teraki, we have developed such a unique radar ML pre-processing that leads to up to 25% more accurate and more robust detections (less sensitive to confidence levels). Regardless of the resolution of the radar, Teraki ML-detection delivers more points per object than CFAR. For instance, Teraki’s approach detects 15% more objects and classification rates improve by up to 25% per available frame and bitrate. The key behind these results is that Teraki ML detection maintains a higher density of points per target when compared to CFAR detections that tend to fade away – as shown in Figure 2
.jpg)
The main benefit of this new approach is that it provides more safety in scenarios where earlier detection of obstacles at far distances is needed. Teraki's radar pre-processing leads to better classification results and impact on its customer's other neural networks and accelerator schemes. Correspondingly, real-time processing performance on selected single-core chipsets is possible, which alleviates the computing requirements by ingesting 4- or 5-bit bitrates instead of 8- or 32bits without compromising the F1-scores.
The increased AI performance brought by Teraki's ML pipeline is a groundbreaking achievement for the AI and automotive community and one of the first of its kind in the industry. As reflected before, radar based L2+ applications demand high accuracy, robustness, and real-time capability requirements. Besides, merely detecting targets will not advance the applications of autonomous driving. Object classification capability is required to distinguish the different types of road users and other elements on the road. This is precisely what Teraki's approach brings to the table. Teraki's ML pipeline efficiently processes and classifies radar signals at the edge, identifying objects with decreased computing capacities and helping to meet the safety concerns of autonomous operations. It essentially allows vehicles to make better decisions, and therefore, more robust AD outcomes and safer cars.
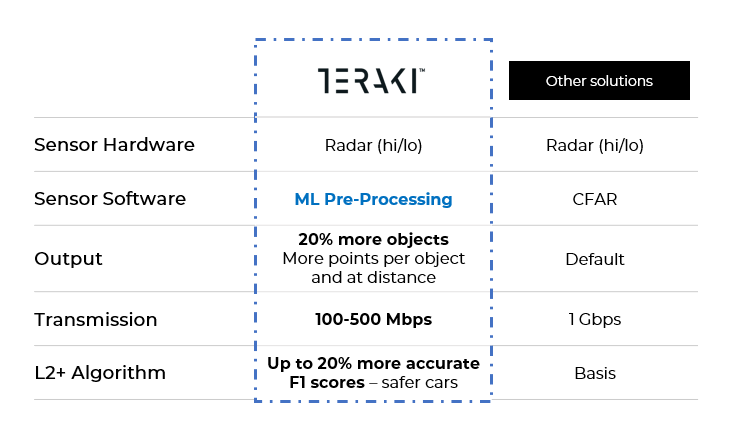
The future of autonomy, today
At Teraki, we have worked extensively for more than six years hand in hand with automotive and semiconductor companies to develop safer autonomous driving applications through efficient pre-processing of sensor data at the edge. Today, we have leveraged all that know-how to offer our customers edge pre-processing for more reliable AD stacks. We believe the future of autonomy is today.
Contact us at info@teraki.com to learn more about our automotive solutions and do not forget to sign up to our monthly newsletter where we will bring you our latest news and developments – sign up here